Nplot Document
In this tutorial, we illustrate the usage of NLopt in various languages via one or two trivial examples.
Example nonlinearly constrained problem
Generic X-Y Plotting. Generic function for plotting of R objects. For more details about the graphical parameter arguments, see par. For simple scatter plots, plot.default will be used. The NPlot library (NPlot.dll) can be downloaded from the NPlot home page. There are different DLLs for the.NET versions 1.1 and 2.0. NPlot's Programming Philosophy When generating a chart using NPlot or any other charting engine, the actual chart must be rendered as a temporary image file on the web server. C# Class NPlot.LinePlot Encapsulates functionality for plotting data as a line chart.

C# Class NPlot.LinePlot Encapsulates functionality for plotting data as a line chart. OxyPlot is a cross-platform plotting library for.NET. The code is licensed under the MIT license. This is a very permissive and corporate friendly license.
As a first example, we'll look at the following simple nonlinearly constrained minimization problem:
subject to , , and
for parameters a1=2, b1=0, a2=-1, b2=1.
The feasible region defined by these constraints is plotted at right: x2 is constrained to lie above the maximum of two cubics, and the optimum point is located at the intersection (1/3, 8/27) where the objective function takes on the value .
(This problem is especially trivial, because by formulating it in terms of the cube root of x2 you can turn it into a linear-programming problem, but we won't do that here.)
In principle, we don't need the bound constraint x2≥0, since the nonlinear constraints already imply a positive-x2 feasible region. However, NLopt doesn't guarantee that, on the way to finding the optimum, it won't violate the nonlinear constraints at some intermediate steps, while it does guarantee that all intermediate steps will satisfy the bound constraints. So, we will explicitly impose x2≥0 in order to ensure that the √x2 in our objective is real.
Note: The objective function here is not differentiable at x2=0. This doesn't cause problems in the examples below, but may cause problems with some other algorithms if they try to evaluate the gradient at x2=0 (e.g. I've seen it cause AUGLAG with a gradient-based solver to fail). To prevent this, you might want to use a small nonzero lower bound instead, e.g. x2≥10−6.
Example in C/C++
To implement the above example in C or C++, we would first do:
to include the NLopt header file as well as the standard math header file (needed for things like the sqrt
function and the HUGE_VAL
constant), then we would define our objective function as:
There are several things to notice here. First, since this is C, our indices are zero-based, so we have x[0]
and x[1]
instead of x1 and x2. The return value of our function is the objective . Also, if the parameter grad
is not NULL
, then we set grad[0]
and grad[1]
to the partial derivatives of our objective with respect to x[0]
and x[1]
. The gradient is only needed for gradient-based algorithms; if you use a derivative-free optimization algorithm, grad
will always be NULL
and you need never compute any derivatives. Finally, we have an extra parameter my_func_data
that can be used to pass additional data to myfunc
, but no additional data is needed here so that parameter is unused.
For the constraints, on the other hand, we will have additional data. Each constraint is parameterized by two numbers a and b, so we will declare a data structure to hold this information:
Then, we implement our constraint function as follows.
The form of the constraint function is the same as that of the objective function. Here, the data
parameter will actually be a pointer to my_constraint_data
(because this is the type that we will pass to nlopt_minimize_constrained
below), so we use a typecast to get the constraint data. NLopt always expects constraints to be of the form myconstraint
(x) ≤ 0, so we implement the constraint x2 ≥ (ax1 + b)3 as the function (ax1 + b)3 − x2. Again, we only compute the gradient if grad
is non-NULL
, which will never occur if we use a derivative-free optimization algorithm.
Now, to specify this optimization problem, we create an 'object' of type nlopt_opt
(an opaque pointer type) and set its various parameters:
Note that we do not need to set an upper bound (nlopt_set_upper_bounds
), since we are happy with the default upper bounds (+∞). To add the two inequality constraints, we do:
Here, the 1e-8
is an optional tolerance for the constraint: for purposes of convergence testing, a point will be considered feasible if the constraint is violated (is positive) by that tolerance (10−8). A nonzero tolerance is a good idea for many algorithms lest tiny errors prevent convergence. Speaking of convergence tests, we should also set one or more stopping criteria, e.g. a relative tolerance on the optimization parameters x:
There are many more possible parameters that you can set to control the optimization, which are described in detail by the reference manual, but these are enough for our example here (any unspecified parameters are set to innocuous defaults). At this point, we can call nlopt_optimize to actually perform the optimization, starting with some initial guess:
nlopt_optimize
will return a negative result code on failure, but this usually only happens if you pass invalid parameters, it runs out of memory, or something like that. (Actually, most of the other NLopt functions also return an error code that you can check if you are paranoid.) (However, if it returns the failure code NLOPT_ROUNDOFF_LIMITED
, indicating a breakdown due to roundoff errors, the minimum found may still be useful and you may want to still use it.) Otherwise, we print out the minimum function value and the corresponding parameters x.
Finally, we should call nlopt_destroy to dispose of the nlopt_opt
object when we are done with it:
Assuming we save this in a file tutorial.c, we would compile and link (on Unix) with:
The result of running the program should then be something like:
That is, it found the correct parameters to about 5 significant digits and the correct minimum function value to about 6 significant digits. (This is better than we specified; this often occurs because the local optimization routines usually try to be conservative in estimating the error.)
Number of evaluations
Let's modify our program to print out the number of function evaluations that were required to obtain this result. First, we'll change our objective function to:
using a global variable count
that is incremented for each function evaluation. (We could also pass a pointer to a counter variable as my_func_data
, if we wanted to avoid global variables.) Then, adding a printf
:
we obtain:
For such a simple problem, a gradient-based local optimization algorithm like MMA can converge very quickly!
Switching to a derivative-free algorithm
We can also try a derivative-free algorithm. Looking at the NLopt Algorithms list, another algorithm in NLopt that handles nonlinear constraints is COBYLA, which is derivative-free. To use it, we just change NLOPT_LD_MMA
('LD' means local optimization, derivative/gradient-based) into NLOPT_LN_COBYLA
('LN' means local optimization, no derivatives), and obtain:
In such a low-dimensional problem, derivative-free algorithms usually work quite well—in this case, it only triples the number of function evaluations. However, the comparison is not perfect because, for the same relative x tolerance of 10−4, COBYLA is a bit less conservative and only finds the solution to 3 significant digits.
To do a fairer comparison of the two algorithms, we could set the x tolerance to zero and ask how many function evaluations each one requires to get the correct answer to three decimal places. We can specify this by using the stopval
termination criterion, which allows us to halt the process as soon as a feasible point attains an objective function value less than stopval
. In this case, we would set stopval
to , replacing nlopt_set_xtol_rel
with the statement:
corresponding to the last line of arguments to nlopt_minimize_constrained
being sqrt(8./27.)+1e-3,
0.0,
0.0,
0.0,
NULL,
0,
0.0
. If we do this, we find that COBYLA requires 25 evaluations while MMA requires 10.
The advantage of gradient-based algorithms over derivative-free algorithms typically grows for higher-dimensional problems. On the other hand, derivative-free algorithms are much easier to use because you don't need to worry about how to compute the gradient (which might be tricky if the function is very complicated).
Example in C++
Although it is perfectly possible to use the C interface from C++, many C++ programmers will find it more natural to use real C++ objects instead of opaque nlopt_opt
pointers, std::vector<double>
instead of arrays, and exceptions instead of error codes. NLopt provides a C++ header file nlopt.hpp
that you can use for this purpose, which simply wraps a C++ object interface around the C interface above.
The equivalent of the above example would then be:
There is no need to deallocate the opt
object; its destructor will do that for you once it goes out of scope. Also, there is no longer any need to check for error codes; the NLopt C++ functions will throw exceptions if there is an error, which you can catch
normally.
Here, we are using the same objective and constraint functions as in C, taking double*
array arguments. Alternatively, you can define objective and constraint functions to take std::vector<double>
arguments if you prefer. (Using std::vector<double>
in the objective/constraint imposes a slight overhead because NLopt must copy the double*
data to a std::vector<double>
, but this overhead is unlikely to be significant in most real applications.) That is, you would do:
Notice that, instead of checking whether grad
is NULL
, we check whether it is empty. (The vector arguments, if non-empty, are guaranteed to be of the same size as the dimension of the problem that you specified.) We then specify these in the same way as before:
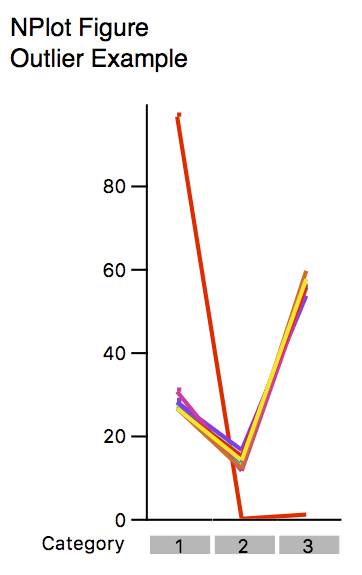
Note that the data pointers passed to these functions must remain valid (or rather, what they point to must remain valid) until you are done with opt
. (It might have been nicer to use shared_ptr
, but I don't like to rely on bleeding-edge language features.)
Instead of passing a separate data pointer, some users may wish to define a C++ function object class that contains all of the data needed by their function, with an overloaded operator()
method to implement the function call. You can easily do this with a two-line helper function. If your function class is MyFunction, then you could define a static member function:
which you would then use e.g. by opt.set_min_objective(MyFunction::wrap, &some_MyFunction)
. Again, you have to make sure that some_MyFunction
does not go out of scope before you are done calling nlopt::opt::optimize
.
To link your program, just link to the C NLopt library (-lnlopt
-lm
on Unix).
Example in Matlab or GNU Octave
To implement this objective function in Matlab (or GNU Octave), we would write a file myfunc.m that looks like:
Notice that we check the Matlab builtin variable nargout
(the number of output arguments) to decide whether to compute the gradient. If we use a derivative-free optimization algorithm below, then nargout
will always be 1 and the gradient need never be computed.

Our constraint function looks similar, except that it is parameterized by the coefficients a and b. We can just add these on as extra parameters, in a file myconstraint.m
:
The equivalent of the nlopt_opt
is just a structure, with fields corresponding to any parameters that we want to set. (Any structure fields that we don't include are equivalent to not setting those parameters, and using the defaults instead). You can get more information on the available parameters by typing help
nlopt_optimize
in Matlab. The equivalent of the C example above is to define an opt
structure by:
We do not need to specify the dimension of the problem; this is implicitly specified by the size of the initial-guess vector passed to nlopt_optimize
below (and must match the sizes of other vectors like opt.lower_bounds
). The inequality constraints are specified as a cell arrayopt.fc
of function handles (and the corresponding tolerances are in an array opt.fc_tol
); notice how we use @(x)
to define an anonymous/inline function in order to pass additional arguments to myconstraint
.
Finally, we call nlopt_optimize
:
nlopt_optimize
returns three things: xopt
, the optimal parameters found; fmin
, the corresponding value of the objective function, and a return code retcode
(positive on success and negative on failure).
The output of the above command is:
(The return code4
corresponds to NLOPT_XTOL_REACHED
, which means it converged to the specified x tolerance.) To switch to a derivative-free algorithm like COBYLA, we just change opt.algorithm
parameter:
Matlab verbose output
It is often useful to print out some status message to see what is happening, especially if your function evaluation is much slower or if a large number of evaluations are required (e.g. for global optimization). You can, of course, modify your function to print out whatever you want. As a shortcut, however, you can set a verbose option in NLopt's Matlab interface by:
If we do this, then running the MMA algorithm as above yields:
This shows the objective function values at each intermediate step of the optimization. As in the C example above, it converges in 11 steps. The COBYLA algorithm requires a few more iterations, because it doesn't exploit the gradient information:
Notice that some of the objective function values are below the minimum of 0.54433 — these are simply values of the objective function at infeasible points (violating the nonlinear constraints).
Example in Python
The same example in Python is:
Notice that the optimize
method returns only the location of the optimum (as a NumPy array), and that the value of the optimum and the result code are obtained by last_optimum_value
and last_optimize_result
values. Like in C++, the NLopt functions raise exceptions on errors, so we don't need to check return codes to look for errors.
The objective and constraint functions take NumPy arrays as arguments; if the grad
argument is non-empty it must be modified in-place to the value of the gradient. Notice how we use Python's lambda
construct to pass additional parameters to the constraints. Alternatively, we could define the objective/constraints as classes with a __call__(self,
x,
grad)
method so that they can behave like functions.
The result of running the above code should be:
finding the same correct optimum as in the C interface (of course). (The return code4
corresponds to nlopt.XTOL_REACHED
, which means it converged to the specified x tolerance.)
Important: Modifying grad
in-place
The grad argument of your objective/constraint functions must be modified in-place. If you use an operation like
however, Python allocates a new array to hold 2*x
and reassigns grad to point to it, rather than modifying the original contents of grad. This will not work. Instead, you should do:
which overwrites the old contents of grad with 2*x
. See also the NLopt Python Reference.
Example in GNU Guile (Scheme)
In GNU Guile, which is an implementation of the Scheme programming language, the equivalent of the example above would be:
Note that the objective/constraint functions take two arguments, x
and grad
, and return a number. x
is a vector whose length is the dimension of the problem; grad is either false (#f
) if it is not needed, or a vector
that must be modified in-place to the gradient of the function.
On error conditions, the NLopt functions throw exceptions that can be caught by your Scheme code if you wish.
Plot Documentation
The heavy use of side-effects here is a bit unnatural in Scheme, but is used in order to closely map to the C++ interface. (Notice that nlopt::
C++ functions map to nlopt-
Guile functions, and nlopt::opt::
methods map to nlopt-opt-
functions that take the opt
object as the first argument.) Of course, you are free to wrap your own Scheme-like functional interface around this if you wish.
Example in Fortran
In Fortran, the equivalent of the C example above would be as follows. First, we would write our functions as:
Notice that that the 'functions' are actually subroutines. This is because it turns out to be hard to call Fortran functions from C or vice versa in any remotely portable way. Therefore:
- In the NLopt Fortran interface, all C functions become subroutines in Fortran, with the return value becoming the first argument.
So, here the first argument val
is used for the return value. Also, because there is no way in Fortran to pass NULL
for the grad
argument, we add an additional need_gradient
argument which is nonzero if the gradient needs to be computed. Finally, the last argument is the equivalent of the void*
argument in the C API, and can be used to pass a single argument of any type through to the objective/constraint functions: here, we use it in myconstraint
to pass an array of two values for the constants a and b.
Then, to run the optimization, we can use the following Fortran program:
There are a few things to note here:
- All
nlopt_
functions are converted intonlo_
subroutines, with return values converted into the first argument. - The '
nlopt_opt
' variableopt
is declared asinteger*8
. (Technically, we could use any type that is big enough to hold a pointer on all platforms;integer*8
is big enough for pointers on both 32-bit and 64-bit machines.) - The subroutines must be declared as
external
. - We
include
'nlopt.f'
in order to get the various constants likeNLOPT_LD_MMA
.
There is no standard Fortran 77 equivalent of C's HUGE_VAL
constant, so instead we just call nlo_get_lower_bounds
to get the default lower bounds (-∞) and then change one of them. In Fortran 90 (and supported as an extension in many Fortran 77 compilers), there is a huge
intrinsic function that we could have used instead:
Example in Julia
Plot Document Verification

The same example in the Julia programming language can be found at the NLopt.jl web page.
List of all members.Detailed Description
A Windows.Forms PlotSurface2D control.Unfortunately it's not possible to derive from both Control and NPlot.PlotSurface2D.
Definition at line 72 of file Windows.PlotSurface2D.cs.Pandas Plot Documentation
Public Member Functions | |
void | Add (IDrawable p, NPlot.PlotSurface2D.XAxisPosition xp, NPlot.PlotSurface2D.YAxisPosition yp, int zOrder) |
Adds a drawable object to the plot surface against the specified axes. | |
void | Add (IDrawable p, int zOrder) |
Adds a drawable object to the plot surface. | |
void | Add (IDrawable p, NPlot.PlotSurface2D.XAxisPosition xp, NPlot.PlotSurface2D.YAxisPosition yp) |
Adds a drawable object to the plot surface against the specified axes. | |
void | Add (IDrawable p) |
Adds a drawable object to the plot surface. | |
void | AddAxesConstraint (AxesConstraint c) |
Add an axis constraint to the plot surface. | |
void | AddInteraction (Interactions.Interaction i) |
Adds and interaction to the plotsurface that adds functionality that responds to a set of mouse / keyboard events. | |
void | CacheAxes () |
Remembers the current axes - useful in interactions. | |
void | Clear () |
Clears the plot and resets to default values. | |
void | CopyDataToClipboard () |
Coppies data in the current plot surface view window to the clipboard as text. | |
void | CopyToClipboard () |
Coppies the chart currently shown in the control to the clipboard as an image. | |
void | DoMouseDown (MouseEventArgs e) |
All functionality of the OnMouseDown function is contained here. | |
void | DoMouseMove (MouseEventArgs e, System.Windows.Forms.Control ctr) |
All functionality of the OnMouseMove function is contained here. | |
void | DoMouseUp (MouseEventArgs e, System.Windows.Forms.Control ctr) |
All functionality of the OnMouseUp function is contained here. | |
void | DoMouseWheel (MouseEventArgs e) |
All functionality of the OnMouseWheel function is containd here. | |
void | DoPaint (PaintEventArgs pe, int width, int height) |
All functionality of the OnPaint method is provided by this function. | |
void | Draw (Graphics g, Rectangle bounds) |
Draws the plot surface on the supplied graphics surface [not the control surface]. | |
delegate void | InteractionHandler (object sender) |
This is the signature of the function used for InteractionOccurred events. | |
void | OriginalDimensions () |
sets axes to be those saved in the cache. | |
PlotSurface2D () | |
Default constructor. | |
delegate void | PreRefreshHandler (object sender) |
This is the signature of the function used for PreRefresh events. | |
void | Print (bool preview) |
Print the chart as currently shown by the control. | |
void | Remove (IDrawable p, bool updateAxes) |
Remove a drawable object from the plot surface. | |
void | RemoveInteraction (Interactions.Interaction i) |
Remove a previously added interaction. | |
Protected Member Functions | |
override void | Dispose (bool disposing) |
Clean up any resources being used. | |
void | OnInteractionOccured (object sender) |
Default function called when plotsurface modifying interaction occured. | |
override void | OnKeyDown (KeyEventArgs e) |
the key down callback | |
override void | OnKeyUp (KeyEventArgs e) |
The key up callback. | |
override void | OnMouseDown (MouseEventArgs e) |
Mouse down event handler. | |
override void | OnMouseMove (MouseEventArgs e) |
MouseMove event handler. | |
override void | OnMouseUp (MouseEventArgs e) |
mouse up event handler. | |
override void | OnMouseWheel (MouseEventArgs e) |
Mouse Wheel event handler. | |
override void | OnPaint (PaintEventArgs pe) |
the paint event callback. | |
void | OnPreRefresh (object sender) |
Default function called just before a refresh happens. | |
Properties | |
bool | AutoScaleAutoGeneratedAxes |
When plots are added to the plot surface, the axes they are attached to are immediately modified to reflect data of the plot. | |
bool | AutoScaleTitle |
Whether or not the title will be scaled according to size of the plot surface. | |
System.ComponentModel.IContainer | components |
bool | DateTimeToolTip |
When true, tool tip will display x value as a DateTime. | |
bool | dateTimeToolTip_ = false |
static PlotContextMenu | DefaultContextMenu |
Gets an instance of a NPlot.Windows.PlotSurface2D.ContextMenu that is useful in typical situations. | |
ArrayList | Drawables |
Gets an array list containing all drawables currently added to the PlotSurface2D. | |
NPlot.PlotSurface2D | Inner |
Allows access to the PlotSurface2D. | |
event InteractionHandler | InteractionOccured |
Event is fired when an interaction happens with the plot that causes it to be modified. | |
ArrayList | interactions_ = new ArrayList() |
KeyEventArgs | lastKeyEventArgs_ = null |
NPlot.Legend | Legend |
Gets or Sets the legend to use with this plot surface. | |
int | LegendZOrder |
Gets or Sets the legend z-order. | |
int | Padding |
Padding of this width will be left between what is drawn and the control border. | |
PhysicalAxis | PhysicalXAxis1Cache |
The physical XAxis1 that was last drawn. | |
PhysicalAxis | PhysicalXAxis2Cache |
The physical XAxis2 that was last drawn. | |
PhysicalAxis | PhysicalYAxis1Cache |
The physical YAxis1 that was last drawn. | |
PhysicalAxis | PhysicalYAxis2Cache |
The physical YAxis2 that was last drawn. | |
IRectangleBrush | PlotBackBrush |
A Rectangle brush used to paint the plot background. | |
System.Drawing.Color | PlotBackColor |
A color used to paint the plot background. | |
System.Drawing.Bitmap | PlotBackImage |
An imaged used to paint the plot background. | |
event PreRefreshHandler | PreRefresh |
Event fired when we are about to paint. | |
NPlot.Windows.PlotSurface2D.PlotContextMenu | RightMenu |
Sets the right context menu. | |
NPlot.Windows.PlotSurface2D.PlotContextMenu | rightMenu_ = null |
bool | ShowCoordinates |
Flag to display a coordinates in a tooltip. | |
System.Drawing.Drawing2D.SmoothingMode | SmoothingMode |
Set smoothing mode for drawing plot objects. | |
string | Title |
The plot surface title. | |
Brush | TitleBrush |
The brush used for drawing the title. | |
Color | TitleColor |
Sets the title to be drawn using a solid brush of this color. | |
Font | TitleFont |
The font used to draw the title. | |
Axis | XAxis1 |
The first abscissa axis. | |
Axis | XAxis2 |
The second abscissa axis. | |
Axis | YAxis1 |
The first ordinate axis. | |
Axis | YAxis2 |
The second ordinate axis. | |
Private Member Functions | |
void | drawDesignMode (Graphics g, Rectangle bounds) |
Draw a lightweight representation of us for design mode. | |
void | DrawHorizontalSelection (Point start, Point end, System.Windows.Forms.UserControl ctr) |
void | InitializeComponent () |
Required method for Designer support - do not modify the contents of this method with the code editor. | |
void | NPlot_PrintPage (object sender, PrintPageEventArgs ev) |
Private Attributes | |
System.Windows.Forms.ToolTip | coordinates_ |
NPlot.PlotSurface2D | ps_ |
System.Collections.ArrayList | selectedObjects_ |
Axis | xAxis1ZoomCache_ |
Axis | xAxis2ZoomCache_ |
Axis | yAxis1ZoomCache_ |
Axis | yAxis2ZoomCache_ |
Classes | |
class | Interactions |
Encapsulates a number of separate 'Interactions'. More... | |
class | PlotContextMenu |
Summary description for ContextMenu. More... |